Machine Learning Data Labeling: Transforming Business Efficiency
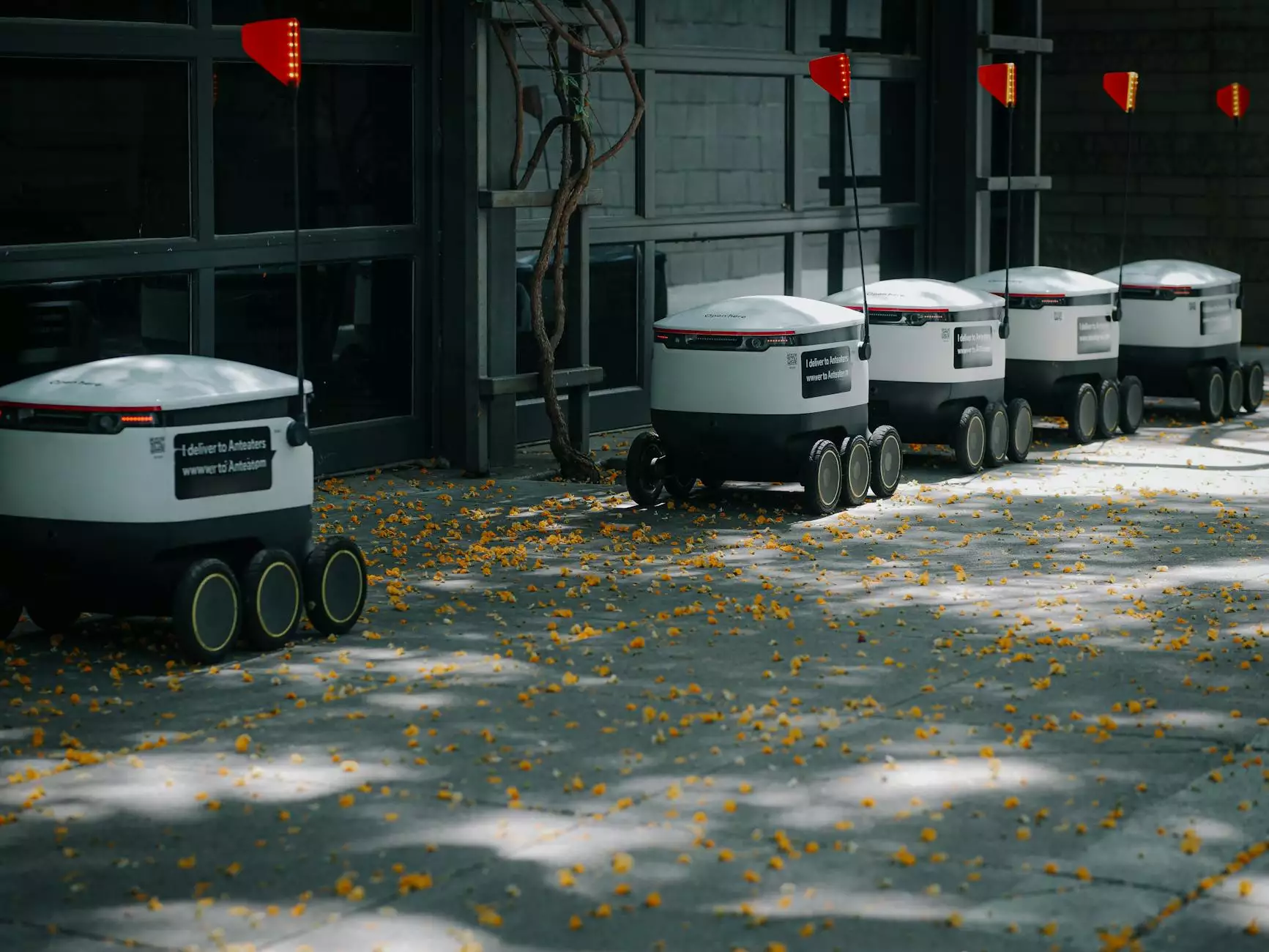
Understanding Machine Learning Data Labeling
Machine learning data labeling is the process of correctly identifying and tagging data to help machines learn. As businesses increasingly rely on artificial intelligence (AI) and machine learning algorithms, the need for accurate data labeling has become paramount. By providing precise annotations, companies can train their models to make sophisticated decisions based on the patterns present in the tagged data.
Why Data Labeling is Crucial for Modern Businesses
The accuracy of a machine learning model correlates directly with the quality of data it learns from. Here are some reasons why data labeling is indispensable for businesses:
- Enhanced Model Performance: High-quality labeled data leads to better predictive accuracy.
- Improved Decision-Making: Data-driven insights allow businesses to make informed strategic choices.
- Competitive Advantage: Businesses utilizing effective data labeling can outperform competitors who neglect this aspect.
- Scalability: Properly labeled datasets can be scaled across different applications and projects.
- Customization: Tailored datasets help in developing specialized models that meet unique business needs.
The Process of Data Annotation
Data annotation involves several key steps that ensure the quality and relevance of the labeled data:
- Data Collection: Gather raw data from various sources such as images, text, or videos.
- Annotation Guidelines: Develop specific guidelines that define how data should be labeled.
- Labeling: Utilize tools and platforms to annotate the data according to established guidelines.
- Quality Assurance: Implement a review process to ensure the accuracy of data labels.
- Data Storage: Properly store labeled data for easy access and integration into machine learning models.
Benefits of Using Data Annotation Tools
Investing in advanced data annotation tools can significantly benefit businesses in numerous ways:
- Increased Efficiency: Automated tools speed up the labeling process, reducing manual effort.
- Consistency: Tools ensure uniformity in labeling, leading to higher data quality.
- Scalability: Businesses can handle larger datasets with ease, meeting growing demands.
- Collaboration: Many platforms enable teams to collaborate seamlessly on annotation projects.
- Customizability: Tailored solutions can meet the specific requirements of different industries.
Choosing the Right Data Annotation Platform
When selecting a data annotation platform, businesses should consider a variety of factors:
- Type of Data Supported: Ensure the platform supports all relevant data types, including images, videos, and text.
- User-Friendliness: The interface should be intuitive to allow easy navigation and efficient workflow.
- Integration Capabilities: Opt for a platform that integrates well with other tools and systems in use.
- Scalability: The platform should accommodate growing data needs without compromising performance.
- Cost-Effectiveness: Analyze the pricing model and select one that fits your budget while delivering good value.
The Impact of Data Labeling on Different Industries
Data labeling has influenced numerous sectors, each benefiting from its implementation:
1. Healthcare
In the healthcare sector, machine learning data labeling is vital for medical imaging, diagnostics, and personalized medicine. Accurate labels improve the training of models that can predict diseases from imaging scans, such as identifying tumors in X-ray or MRI scans.
2. Finance
The finance industry utilizes labeled data for fraud detection and risk assessment. Machine learning models can analyze transaction data to identify anomalies and prevent fraudulent activities.
3. Retail
Retailers leverage data labeling for customer segmentation, personalized marketing, and inventory management. By understanding customer behavior through labeled data, businesses can enhance customer experiences and optimize inventory levels.
4. Automotive
In the automotive industry, data labeling is crucial for developing self-driving car technologies. Labeled datasets are used to train models that recognize objects, road signs, and hazards on the road.
5. E-commerce
E-commerce platforms benefit from data labeling in enhancing search algorithms, recommender systems, and improving user experience through personalized content delivery.
Future Trends in Machine Learning Data Labeling
As technology evolves, several trends are likely to shape the future of machine learning data labeling:
- AI-Powered Annotation: Automated labeling processes will become more intelligent, reducing the need for human intervention.
- Crowdsourced Annotations: Platforms may utilize the crowd's input to quickly and efficiently label vast datasets.
- Real-time Data Labeling: Systems that allow immediate labeling during data collection will gain traction.
- Enhanced Quality Control: Advanced checks and balances will be implemented to ensure data labeling accuracy.
- Integration with Other Technologies: Data labeling tools will blend with emerging technologies like augmented reality (AR) and virtual reality (VR) for enhanced applications.
Conclusion
In summary, machine learning data labeling is not just a technical necessity but a strategic imperative for businesses aiming to innovate and remain competitive. As we navigate through an increasingly data-driven world, understanding and investing in effective data labeling solutions like those provided by KeyLabs.ai can set businesses on a path to sustainable growth and efficiency. The future of data labeling lies in harnessing advanced tools, implementing best practices, and recognizing the irreplaceable value of precise data annotations in business development.
© 2023 KeyLabs.ai. All rights reserved.